1. Introduction: The Analytics Revolution in Investment Management
In the high-stakes world of investment management, where fortunes are made and lost in the blink of an eye, a new champion has emerged: investment analytics. But what if I told you that this game-changer is not just reshaping the financial landscape – it's completely revolutionizing it?
Welcome to the cutting edge of finance, where big data meets Wall Street, and algorithms dance with market trends. Investment analytics is not just a tool; it's a fundamental shift in how we understand, predict, and navigate the complex world of investments. Whether you're a seasoned fund manager, a curious individual investor, or a fintech enthusiast, this comprehensive guide will take you on a journey through the transformative power of analytics in the investment world.
But here's the million-dollar question: Are you ready to harness this power and stay ahead in an increasingly data-driven financial ecosystem? Or will you be left behind, relying on outdated methods in a rapidly evolving market?
Buckle up as we dive deep into the world of investment analytics, exploring everything from AI-powered algorithms to ethical considerations in data-driven investing. This isn't just about understanding numbers; it's about gaining a competitive edge in one of the world's most dynamic industries. Are you prepared to revolutionize your approach to investment? Let's begin our exploration!
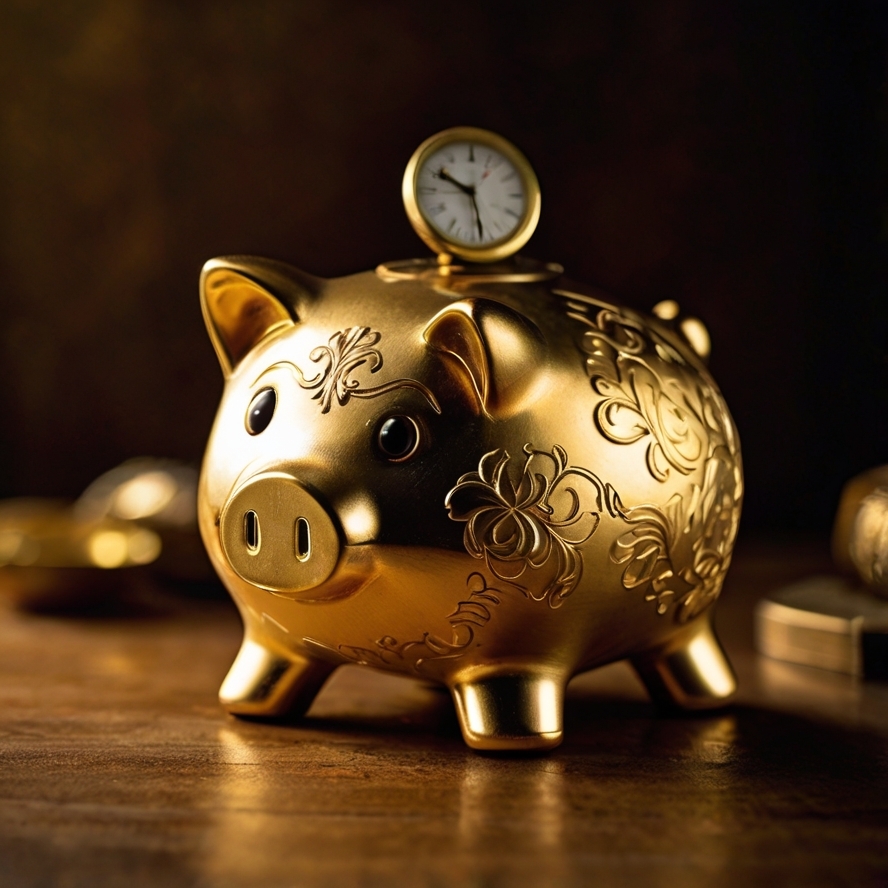
2. Understanding Investment Analytics: Definition and Key Components
At its core, investment analytics is the science of using data analysis, statistical modeling, and machine learning techniques to gain insights and make informed investment decisions. But it's so much more than crunching numbers. Let's break down the key components:
Data Collection and Management
- Traditional financial data (price, volume, financial statements)
- Alternative data (satellite imagery, social media sentiment, web scraping)
- Real-time market data feeds
Data Analysis Techniques
- Statistical analysis
- Machine learning algorithms
- Natural Language Processing (NLP) for unstructured data
Predictive Modeling
- Forecasting market trends
- Risk assessment models
- Asset allocation optimization
Visualization and Reporting
- Interactive dashboards
- Real-time performance metrics
- Customized reporting for different stakeholders
Investment analytics is not just about having access to data; it's about transforming that data into actionable insights. It's the bridge between raw information and informed decision-making, allowing investors to see patterns, predict trends, and manage risks with unprecedented precision.
3. The Evolution of Investment Analytics: From Spreadsheets to AI
The journey of investment analytics is a testament to the rapid advancement of technology in finance. Let's trace this exciting evolution:
1. The Spreadsheet Era (1980s-1990s)
- Introduction of personal computers and spreadsheet software
- Basic financial modeling and data organization
- Limited to manual data entry and simple calculations
2. The Rise of Quantitative Analysis (1990s-2000s)
- Development of sophisticated financial models
- Introduction of statistical software packages
- Emergence of algorithmic trading
3. Big Data Revolution (2000s-2010s)
- Explosion of available data sources
- Development of powerful data processing tools
- Rise of cloud computing for data storage and analysis
4. The AI and Machine Learning Era (2010s-Present)
- Integration of AI and machine learning in investment strategies
- Real-time data analysis and decision-making
- Predictive analytics for market forecasting
5. The Future: Quantum Computing and Beyond
- Potential for quantum computers to revolutionize complex financial modeling
- Integration of blockchain technology for secure and transparent analytics
- Advancements in natural language processing for sentiment analysis
This evolution has not just changed the tools we use; it has fundamentally altered how we approach investment decision-making. From manual calculations to AI-powered predictive models, investment analytics has become increasingly sophisticated, offering deeper insights and faster decision-making capabilities than ever before.
4. Fundamental Analysis in the Age of Big Data
Fundamental analysis, the cornerstone of value investing, has undergone a dramatic transformation in the age of big data and advanced analytics. Let's explore how technology is enhancing this traditional approach:
Enhanced Data Sources
- Real-time financial statement analysis
- Integration of alternative data (e.g., satellite imagery for retail foot traffic)
- Social media sentiment analysis for brand perception
Automated Ratio Calculations
- Instant computation of complex financial ratios
- Historical trend analysis with a click of a button
- Peer comparison across multiple metrics simultaneously
Natural Language Processing (NLP)
- Automated analysis of earnings call transcripts
- Sentiment analysis of news articles and regulatory filings
- Identification of key themes and trends in company communications
Predictive Modeling
- Machine learning models for earnings forecasts
- AI-driven scenario analysis for economic impacts
- Pattern recognition for identifying potential value traps
While the principles of fundamental analysis remain the same, the tools and techniques have evolved dramatically. Investors can now process vast amounts of information quickly, uncover hidden insights, and make more informed decisions. However, it's crucial to remember that technology is an enhancer, not a replacement for human judgment in fundamental analysis.
5. Technical Analysis: Advanced Charting and Pattern Recognition
Technical analysis has come a long way from simple line charts and moving averages. In the era of investment analytics, it has evolved into a sophisticated discipline leveraging cutting-edge technology. Here's how analytics is revolutionizing technical analysis:
Advanced Charting Techniques
- Interactive and customizable charts with multiple data overlays
- 3D and holographic visualizations for complex data relationships
- Real-time updating and streaming charts for live analysis
Automated Pattern Recognition
- AI-powered identification of chart patterns (e.g., head and shoulders, cup and handle)
- Machine learning algorithms for detecting emerging patterns
- Quantification of pattern reliability based on historical data
Multi-Timeframe Analysis
- Simultaneous analysis across multiple timeframes
- Identification of confluences and divergences between timeframes
- Fractal analysis for pattern recognition across scales
Sentiment Integration
- Incorporation of social media sentiment into technical indicators
- Analysis of order flow and market depth for short-term predictions
- Integration of news sentiment with price action analysis
These advancements have made technical analysis more powerful and accessible than ever before. However, it's important to note that while technology can identify patterns and trends, interpretation and decision-making still require human expertise and judgment.
6. Quantitative Analysis: Harnessing the Power of Algorithms
Quantitative analysis, or "quant" strategies, have become increasingly sophisticated with the advent of advanced analytics. Let's explore how algorithms are reshaping this field:
Advanced Statistical Models
- Time series analysis for forecasting market movements
- Monte Carlo simulations for risk assessment
- Factor models for identifying key drivers of returns
Machine Learning Algorithms
- Neural networks for pattern recognition in market data
- Reinforcement learning for optimizing trading strategies
- Ensemble methods combining multiple models for robust predictions
High-Frequency Trading (HFT)
- Microsecond-level trade execution based on market microstructure
- Algorithmic strategies for market making and arbitrage
- Real-time risk management and position adjustments
Natural Language Processing (NLP) in Quant Strategies
- Sentiment analysis of news and social media for trading signals
- Automated analysis of earnings reports and economic indicators
- Topic modeling for identifying emerging market themes
Quantitative analysis has evolved from simple statistical models to complex, AI-driven systems that can process vast amounts of data in real-time. While these strategies offer powerful insights and trading capabilities, they also come with their own set of challenges, including model risk and the need for constant adaptation to changing market conditions.
7. Risk Analysis and Management: Predictive Modeling for Safer Investments
In the world of investments, understanding and managing risk is paramount. Advanced analytics has transformed risk management from a reactive to a proactive discipline. Here's how:
Comprehensive Risk Modeling
- Value at Risk (VaR) calculations using Monte Carlo simulations
- Stress testing portfolios against various market scenarios
- Tail risk analysis for assessing extreme market events
Real-Time Risk Monitoring
- Continuous assessment of portfolio risk factors
- Automated alerts for breaches of risk thresholds
- Dynamic risk adjustment based on market conditions
Predictive Risk Analytics
- Machine learning models for forecasting potential risk events
- Sentiment analysis to gauge market risk appetite
- Scenario planning based on geopolitical and economic factors
Integrated Risk Management
- Holistic view of risk across different asset classes and strategies
- Correlation analysis to identify hidden risk dependencies
- Risk attribution to understand sources of portfolio risk
These advanced risk management techniques allow investors to not only better understand their current risk exposure but also anticipate and prepare for potential future risks. However, it's crucial to remember that no model can predict every possible scenario, and human oversight remains essential in interpreting and acting on risk analytics.
8. Portfolio Analytics: Optimizing Asset Allocation and Performance
Portfolio analytics has evolved significantly, offering investors powerful tools to optimize their asset allocation and track performance. Here's how analytics is revolutionizing portfolio management:
Advanced Asset Allocation Models
- Multi-factor optimization considering risk, return, and correlation
- Dynamic asset allocation adjusting to market conditions
- Incorporation of alternative assets for enhanced diversification
Performance Attribution
- Granular analysis of returns by sector, style, and individual securities
- Risk-adjusted performance metrics (e.g., Sharpe ratio, Jensen's alpha)
- Peer group comparison and benchmarking
Scenario Analysis and Stress Testing
- Monte Carlo simulations for projecting future portfolio outcomes
- Historical scenario analysis to assess portfolio resilience
- Custom stress tests based on specific market events or hypothetical scenarios
Tax Efficiency and Rebalancing
- Automated tax-loss harvesting strategies
- Intelligent rebalancing considering tax implications and transaction costs
- After-tax return optimization
These advanced portfolio analytics tools enable investors to construct more robust, efficient portfolios tailored to their specific goals and risk tolerance. However, it's important to remember that while analytics can provide valuable insights, the final investment decisions should always consider broader market context and individual investor circumstances.
9. Machine Learning and AI in Investment Decision Making
The integration of Machine Learning (ML) and Artificial Intelligence (AI) into investment decision-making processes is perhaps one of the most exciting developments in finance. Here's how these technologies are being applied:
Predictive Analytics
- Forecasting market trends and asset prices
- Identifying potential market anomalies and arbitrage opportunities
- Predicting company earnings and economic indicators
Natural Language Processing (NLP)
- Analyzing news articles, social media, and company reports for sentiment
- Extracting key information from financial documents
- Real-time monitoring of market-moving news and events
Algorithmic Trading
- Developing and optimizing trading strategies
- High-frequency trading based on real-time market data
- Automated execution of trades to minimize market impact
Risk Management
- Identifying potential risks in portfolios
- Stress testing under various market scenarios
- Fraud detection and prevention
While ML and AI offer powerful capabilities, it's crucial to remember that they are tools to augment human decision-making, not replace it entirely. The most successful approaches often combine the computational power of AI with human expertise and intuition.
10. Alternative Data Sources: Gaining an Edge in the Market
In the quest for alpha, investors are increasingly turning to alternative data sources to gain unique insights. Here's how alternative data is revolutionizing investment analytics:
Types of Alternative Data
- Satellite imagery (e.g., tracking retail parking lot traffic)
- Credit card transaction data
- Mobile phone location data
- Social media sentiment
- Web scraping of online prices and product listings
Applications in Investment
- Predicting company revenues before official reports
- Assessing consumer behavior and trends
- Monitoring supply chain disruptions
- Evaluating brand health and customer sentiment
Challenges in Using Alternative Data
- Data quality and reliability concerns
- Legal and ethical considerations
- Integration with traditional data sources
- Extracting meaningful signals from noise
While alternative data can provide a competitive edge, it's important to approach it with caution. Proper due diligence, robust data processing techniques, and careful interpretation are crucial for effectively leveraging these novel data sources.
11. Real-Time Analytics: Responding to Market Changes on the Fly
In today's fast-paced markets, the ability to analyze and react to information in real-time is crucial. Here's how real-time analytics is transforming investment decision-making:
Live Market Data Processing
- Streaming analytics of tick-by-tick market data
- Real-time calculation of complex indicators and signals
- Instant portfolio valuation and risk assessment
Automated Trading Systems
- High-frequency trading algorithms
- Dynamic adjustment of trading strategies
- Automated risk management and position sizing
Real-Time News and Social Media Analysis
- Instant processing of breaking news and its potential market impact
- Real-time sentiment analysis from social media feeds
- Automated alerts for significant events or trends
Challenges and Considerations
- Ensuring data accuracy and reliability in real-time environments
- Managing the computational demands of real-time processing
- Balancing speed with thoughtful decision-making
While real-time analytics offers the potential for rapid response to market changes, it's important to implement robust systems and safeguards to prevent knee-jerk reactions based on noisy or misleading short-term data.
12. Ethical Considerations and Regulatory Challenges in Investment Analytics
As investment analytics becomes more sophisticated, it raises important ethical and regulatory questions. Here are key considerations:
Data Privacy and Security
- Ensuring compliance with data protection regulations (e.g., GDPR)
- Protecting sensitive financial information
- Ethical use of alternative data sources
Algorithmic Bias
- Addressing potential biases in AI and ML models
- Ensuring fairness in automated investment decisions
- Transparency in model inputs and decision-making processes
Market Manipulation Concerns
- Preventing the use of analytics for market abuse
- Regulatory challenges in high-frequency trading
- Ensuring fair access to market-moving information
Regulatory Compliance
- Adapting to evolving regulations on AI and data use in finance
- Ensuring auditability of algorithmic trading systems
- Balancing innovation with regulatory requirements
As the field of investment analytics continues to evolve, it's crucial for practitioners to stay ahead of ethical considerations and regulatory requirements, ensuring that technological advancements are leveraged responsibly and fairly.
13. Building an Analytics-Driven Investment Organization
Transforming into an analytics-driven investment organization requires more than just implementing new technologies. It involves a cultural shift and strategic approach:
Developing a Data-Centric Culture
- Fostering a mindset of data-driven decision making
- Encouraging curiosity and continuous learning
- Breaking down silos between teams and departments
Building the Right Team
- Hiring data scientists and quantitative analysts
- Upskilling existing staff in data analytics
- Balancing technical expertise with domain knowledge
Implementing Robust Data Infrastructure
- Investing in scalable data storage and processing capabilities
- Ensuring data quality and governance
- Implementing cybersecurity measures
Fostering Collaboration
- Creating cross-functional teams of analysts, portfolio managers, and technologists
- Establishing processes for sharing insights across the organization
- Encouraging a culture of experimentation and innovation
Building an analytics-driven investment organization is an ongoing process that requires commitment from leadership, investment in technology and talent, and a willingness to adapt to rapidly changing market conditions.
14. The Future of Investment Analytics: Trends and Predictions
As technology continues to evolve, so too will the field of investment analytics. Here are some trends and predictions for the future:
Quantum Computing
- Potential for solving complex optimization problems
- Enhanced cryptography for secure data transmission
- Faster processing of vast datasets
Explainable AI
- Development of more transparent AI models
- Increased trust and adoption of AI in investment decision-making
- Better alignment with regulatory requirements
Advanced Natural Language Processing
- More sophisticated analysis of unstructured data
- Real-time processing of global news and social media
- Improved sentiment analysis and trend prediction
Decentralized Finance (DeFi) Analytics
- Tools for analyzing blockchain-based financial products
- Integration of cryptocurrency data into traditional financial models
- New metrics for assessing DeFi risks and opportunities
While these trends offer exciting possibilities, it's important to approach them with a critical eye, considering both their potential benefits and challenges in practical application.
15. Case Studies: Successful Implementation of Analytics in Investment Firms
Let's examine two real-world examples of how investment firms have successfully leveraged analytics:
Case Study 1: Quantitative Hedge Fund
A leading quantitative hedge fund implemented a machine learning-based strategy for predicting short-term price movements in highly liquid stocks. By combining traditional market data with alternative data sources like satellite imagery and social media sentiment, they were able to:
- Improve prediction accuracy by 15%
- Reduce portfolio volatility by 20%
- Achieve consistent alpha generation even in turbulent market conditions
Case Study 2: Large Asset Management Firm
A global asset management firm developed an AI-powered risk management system that integrates real-time market data, economic indicators, and geopolitical events. This system enabled them to:
- Identify potential risks before they materialized
- Optimize portfolio allocations dynamically
- Improve client communication with data-driven insights
These case studies highlight the tangible benefits of advanced analytics in investment management, demonstrating how data-driven approaches can lead to improved performance and risk management.
16. Balancing Human Intuition and Data-Driven Insights in Investing
While analytics provides powerful tools for investment decision-making, the role of human judgment remains crucial. Here's how to strike the right balance:
Complementary Strengths
- Analytics: Processing vast amounts of data, identifying patterns
- Human Intuition: Understanding context, adapting to new situations
Overcoming Biases
- Use analytics to challenge cognitive biases
- Apply human judgment to question model assumptions
Continuous Learning
- Regularly update models based on new data and market conditions
- Encourage ongoing education in both analytics and fundamental investing
Ethical Considerations
- Use human oversight to ensure ethical use of analytics
- Consider broader societal impacts of investment decisions
The most successful investment strategies of the future will likely be those that effectively combine the computational power of analytics with the nuanced understanding and adaptability of human experts.
17. Conclusion: Embracing Analytics for Competitive Advantage in Investment Management
As we've explored throughout this comprehensive guide, investment analytics is not just a trend – it's a fundamental shift in how the financial world operates. From AI-powered predictive models to alternative data sources, the tools and techniques of investment analytics are reshaping every aspect of the investment process.
Key takeaways include:
- The importance of integrating multiple data sources and analytical techniques
- The growing role of AI and machine learning in investment decision-making
- The need for robust risk management and ethical considerations in data-driven investing
- The value of balancing advanced analytics with human expertise and intuition
- The exciting future prospects, including quantum computing and explainable AI
As we look to the future, it's clear that those who can effectively harness the power of analytics will have a significant competitive advantage in the investment world. However, success will not just be about having the most advanced technology or the biggest data sets. It will be about asking the right questions, interpreting results wisely, and applying insights in ways that align with investment goals and ethical standards.
For professionals in the investment industry, continuous learning and adaptation will be key. Embrace the power of analytics, but don't lose sight of the fundamental principles of sound investing. For individual investors, understanding these trends can help you make more informed decisions about where to entrust your capital.
The world of investment analytics is evolving rapidly, offering both exciting opportunities and significant challenges. By staying informed, adaptable, and thoughtful in our approach, we can navigate this new landscape and potentially unlock unprecedented insights and returns in the ever-changing world of investments.